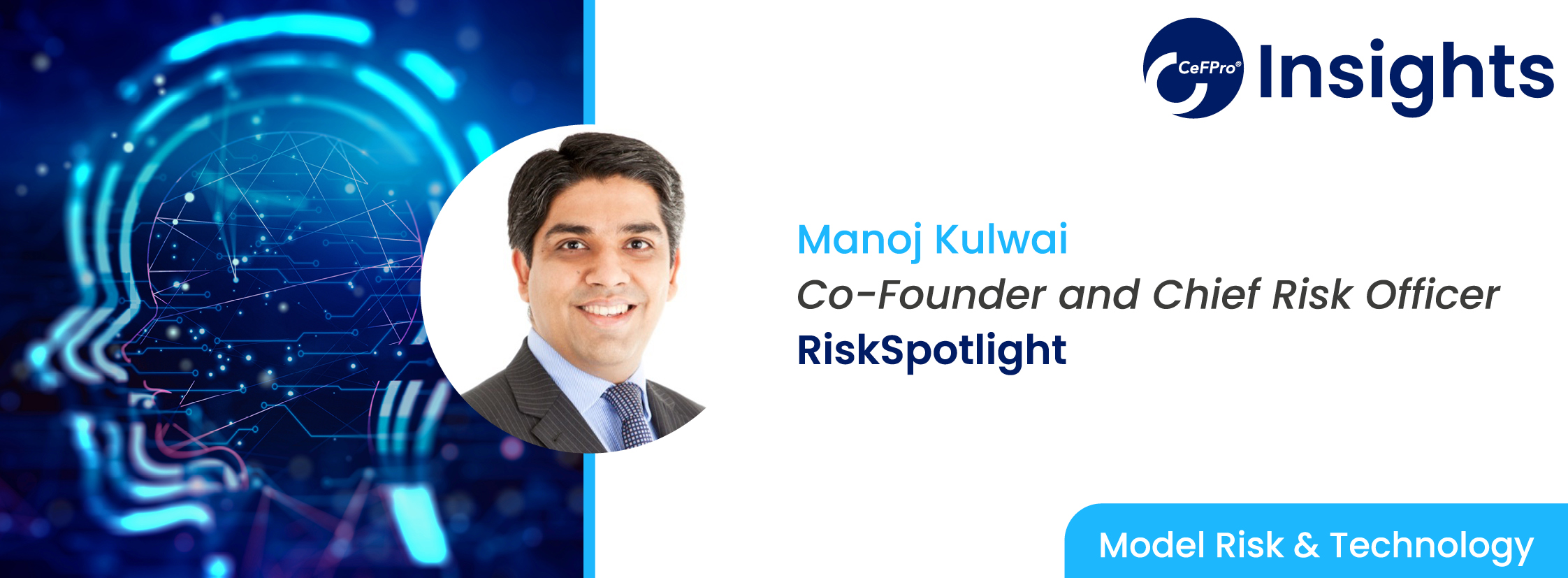
Reviewing practical non-financial risk (NFR) management use cases utilizing Generative AI
Manoj Kulwal, Co-Founder and Chief Risk Officer, RiskSpotlight
Below is an insight into what can be expected from Manoj’s session at Risk Evolve 2024.
The views and opinions expressed in this article are those of the thought leader as an individual, and are not attributed to CeFPro or any particular organization.
Can you share some examples of practical NFR management use cases where Generative AI has been successfully implemented, and what outcomes have been observed?
The introduction of Generative AI into the management of Non-Financial Risks (NFR) within the financial services sector marks a significant advancement in risk management practices. At RiskSpotlight, we have created a library of 100+ risk and compliance use cases where Generative AI can add significant benefits. Let me share three use cases as key examples.
Enhancing Control Documentation Quality
A pervasive issue across many financial institutions is the quality of control documentation. Poorly documented controls not only hinder compliance efforts but also obscure the understanding of risk mitigation strategies in place. Generative AI can provide significant benefits for this use case. These technologies are capable of rigorously assessing existing control documentation against industry best practices, pinpointing discrepancies, and areas lacking in detail or clarity. More impressively, Generative AI can go a step further by generating revised control documentation that adheres to control documentation best practices. The implications of this are profound, offering not just a substantial reduction in the time and effort expended by first-line control owners but also a marked enhancement in the quality of control documentation.
Identifying Risk and Control Gaps
Another use case where Generative AI demonstrates its value is in the identification of risk or control gaps. Despite the diligent efforts of financial firms to catalog risks associated with their processes, products, and IT systems, the complexity and dynamic nature of the business context mean that gaps can and do arise. Utilizing Generative AI tools for a comprehensive analysis of the currently identified risks offers a fresh perspective, revealing any overlooked or emerging risks. Similarly, these AI tools can evaluate the controls mapped to specific risks, identifying missing key controls that are critical for mitigating identified risks. This capability is instrumental in closing gaps within the risk register and ensuring a robust and comprehensive risk analysis framework is in place, significantly minimizing potential exposure.
Facilitating Risk and Control Assessments
The third use case centers on the assessment of risks and controls, a task traditionally characterized by its reliance on the analysis of diverse types of information, including recent loss events, key risk indicators, the status of open issues and remediation actions, as well as recent audit findings. Generative AI’s ability to analyze, interpret, and summarize this broad spectrum of information presents a significant leap forward. It enables a more informed, nuanced, and efficient assessment process, enhancing the ability of risk management professionals to make decisions based on a holistic view of the risk landscape.
What is the current landscape of Generative AI uses across risk management, and how are organizations leveraging this technology to enhance their risk management practices?
Many financial institutions are currently exploring Generative AI’s capabilities, primarily focusing on value creation through the development of innovative products and services. A noteworthy example is Morgan Stanley’s deployment of a Generative AI-powered wealth management advisor. This application underscores the technology’s potential to revolutionize customer engagement and service delivery, showcasing how Generative AI can transcend traditional operational roles to drive business growth and enhance client experiences.
Despite the promising applications of Generative AI, its novelty has prompted a cautious approach from most financial firms. The primary concern revolves around the inherent risks of widely adopting new technology, leading to a tentative stance where its use is often restricted. Recognizing these apprehensions, Generative AI vendors have begun to offer enterprise versions of their models. These tailored solutions provide firms with enhanced control over data usage and user interactions with the models, addressing key security and privacy concerns. As firms gain more confidence in managing the associated risks, it’s anticipated that the upcoming 6 to 12 months will see a broader adoption of Generative AI technologies, marking a significant shift in the industry’s approach to leveraging these tools.
The current focus of 2nd line Risk teams largely gravitates towards mitigating risks introduced by Generative AI, with less attention paid to exploiting its potential to enhance risk management practices. RiskSpotlight is conducting interactive Generative AI sessions with 2nd line risk teams globally to raise awareness on the productivity benefits of these technologies for the risk and compliance activities. We are advising firms to embark on pilot projects targeting 3-4 complex use cases over the next six months. Such initiatives are invaluable, furnishing firms with critical insights into both the risks and the operational efficiencies that Generative AI can deliver. These pilot studies serve as a pragmatic approach, enabling firms to meticulously assess the technology’s impact, refine their strategies for broader deployment, and fully embrace Generative AI’s capabilities within their risk management frameworks.
How does the integration of Generative AI enhance the productivity and quality of risk management processes, and what are the benefits to firms?
Non-financial risk management inherently involves the generation and management of substantial volumes of textual data, including risk descriptions, loss event narratives, issue summaries, audit findings, and control test results. Traditional methods of processing and analyzing this data are not only time-consuming but also prone to human error, given the sheer volume and complexity of the information involved. With its capacity to read, understand, and analyze large datasets, Generative AI can sift through the textual data, identifying patterns, insights, and anomalies that might elude even the most diligent human analysts. This capability ensures that no critical piece of information is overlooked, thereby enhancing the decision-making process and risk insight generation. Let me share two examples: –
– If a firm has 5,000 controls currently documented in its control library but is not satisfied with the quality of the documented controls, then it can utilise Generative AI to read documentation of all 5,000 controls and suggest enhancements that should be made to align the control documentation with industry best practices. This task will require many months of a large team of humans to manually read all the control descriptions and suggest enhancements. However, Generative AI can perform this same analysis in few hours.
– If a firm has documented 200 key business processes and the process owners have identified risks associated with each process. However, the risk team is unsure whether all the key risks have been identified for these processes. It will take many weeks for a team of humans to review the risks of all 200 processes to identify gaps. But Generative AI can perform this same analysis in few hours.
The above two examples highlight not only the significant productivity benefits but will also significantly increase the quality of risk and control information.
Generative AI can provide following benefits: –
- Increased productivity by automating risk management activities involving analysis of large amount of risk related content.
- Enhanced quality by utilising the risk management knowledge and expertise provided by the advanced Generative AI models.
- Cost efficiency by fast-tracking analysis of large amounts of risk content and reducing the number of internal/external human experts needed to perform such analysis.
- Improved risk insights by utilising the capabilities of Generative AI models for analysing large amount of text content to produce insightful summaries and information gaps.
What key implementation topics should firms consider for implementing Generative AI across risk management processes?
Firms should consider following implementation topics: –
Integrating Generative AI with GRC Applications
GRC platforms serve as the repositories of a wealth of risk-related information, encapsulating everything from risk assessments and control data to compliance reports and audit findings. While these applications are rich in data, the quality and actionable intelligence derived from this data can vary. By leveraging the API capabilities of leading Generative AI tools, firms can inject advanced analytical and content-generation functionalities directly into their GRC applications. This integration enables the Generative AI to process, analyze, and refine the vast amounts of textual data stored within GRC applications, enhancing the quality, relevance, and utility of the risk information.
Such an integration not only streamlines the identification of risk exposures and the assessment of control effectiveness but also enriches the risk documentation with insights aligned with the latest industry standards and practices. The ability of Generative AI to generate comprehensive reports, risk assessments, and even recommendations for control optimization transforms the way firms approach their risk management tasks, making them more efficient, informed, and agile in their decision-making processes.
Adopting a Phased Approach
To effectively leverage Generative AI in risk management, firms are advised to adopt a phased approach, focusing on a select number of key use cases within each phase. Typically, each phase could span 3-4 months and concentrate on 2-3 significant risk management areas where Generative AI has the potential to deliver substantial benefits. This methodical approach allows firms to meticulously plan, execute, and evaluate the impact of Generative AI on each selected use case, ensuring that the implementation is both strategic and manageable.
Attempting to deploy Generative AI across too broad a spectrum of use cases simultaneously can dilute focus, stretch resources thin, and potentially hinder the ability to demonstrate clear benefits. Conversely, a phased approach enables firms to build on the successes and learnings from each stage, gradually expanding the application of Generative AI across the risk management domain. This not only ensures a more controlled and effective integration of the technology but also allows for the iterative refinement of strategies based on real-world outcomes and feedback.
Utilise the Enterprise versions of Generative AI models
We advocate for firms to consider the adoption of Enterprise versions of Generative AI models, given their superior knowledge depth and enhanced reasoning capabilities. While it is common for Generative AI vendors to provide a complimentary version of their models, our extensive research has revealed a discernible disparity in quality between these free offerings and their paid counterparts, particularly in the context of risk management activities. The outcomes derived from the complimentary models are often subpar, which could inadvertently lead to misguided decisions regarding the adoption of Generative AI technologies. Consequently, relying on the performance of free models might not only result in inaccurate assessments of Generative AI’s potential but also postpone the realization of its considerable benefits. Thus, to fully harness the transformative power of Generative AI within risk management processes, firms should only consider investing in the Enterprise versions of these models.
Customizing and Training of AI models
To maximize the efficacy and relevance of Generative AI within their operations, firms should prioritize the utilization of models that offer the flexibility to be customized and trained specifically on their unique risk management frameworks, policies, and the distinct risk content that underpins their business operations. This approach ensures that the outputs generated by the AI are inherently aligned with the firm’s specific risk context, thereby providing insights and responses that are far more pertinent and immediately actionable than generic risk management outputs. By doing so, employees benefit from AI-driven analyses and recommendations that are directly applicable to their day-to-day risk management challenges, significantly enhancing the value of these AI capabilities. Tailoring Generative AI in this manner not only amplifies its utility across the organization but also fortifies the firm’s overall risk posture by embedding deep, context-aware intelligence into the fabric of its risk management processes.
Cross-functional Collaboration
Cross-functional collaboration is essential when integrating Generative AI into risk management processes, bridging the gap between risk management teams and other departments such as IT, compliance, and operations. This collaborative approach ensures that Generative AI initiatives are aligned with the broader organizational goals and adhere to regulatory requirements. By bringing together diverse perspectives and expertise, firms can tailor AI solutions to address specific risk management needs effectively while ensuring these solutions fit seamlessly into the existing technological and operational infrastructure. Moreover, such collaboration fosters a unified understanding of the potential and limitations of Generative AI across the organization, facilitating smoother implementation, higher adoption rates, and more effective use of AI technologies. Ultimately, cross-functional collaboration not only enhances the strategic deployment of Generative AI but also reinforces the organization’s collective ability to manage risks in a cohesive and comprehensive manner.
Which Generative AI solutions do you suggest financial services firms to explore?
In the landscape of Generative AI solutions tailored for financial services, the Enterprise and Teams editions of ChatGPT stand out as the best choice currently for addressing risk and compliance use cases. These versions not only provide access to advanced Generative AI models but also offer the flexibility of integrating these capabilities into firms’ existing risk management applications via ChatGPT API. This approach not only reduces the necessity for extensive licensing but also grants firms enhanced oversight on employee interaction with the AI models, ensuring both efficiency and compliance.
Microsoft Copilot, although built upon the ChatGPT framework and designed to seamlessly integrate with Microsoft’s suite of applications, has been observed to produce outputs that, according to our research, do not match the quality levels of the direct ChatGPT models.
However, the AI domain is rapidly evolving, with several models emerging as potential front-runners in the near future. While currently, they may not rival ChatGPT in output quality, their development trajectory suggests they will become significant contenders. Financial services firms should consider following models in their decision making:
- Claude (by Anthropic) – Known for its ethical AI focus, offering promising advancements.
- Gemini (by Google) – With Google’s vast data and AI expertise, Gemini is expected to make significant leaps.
- Perplexity (by Perplexity AI) – Aims at providing intuitive and context-aware AI solutions.
- LLaMa (by Meta) – Meta’s foray into the Generative AI space, focusing on versatile applications.
- StableLM (by Stability AI) – Emphasizes stability and reliability in its AI offerings.
- Falcon (by Technology Innovation Institute) – Represents innovative approaches to AI, leveraging cutting-edge research.