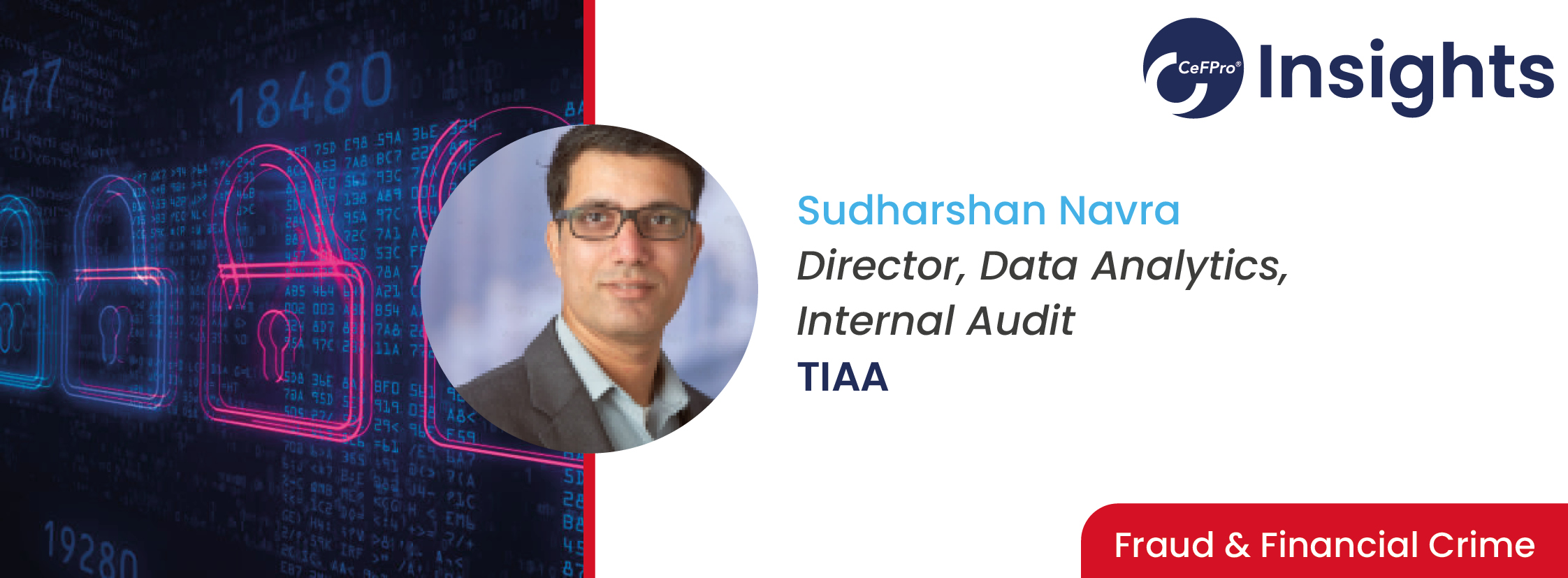
Leveraging the use of technology including AI and machine learning to optimize programs and enhance transaction monitoring.
Sudharshan Navra, Director, Data Analytics, Internal Audit, TIAA
Below is an insight into what can be expected from Sudharshan’s session at Fraud & Financial Crime USA 2023.
The views and opinions expressed in this article are those of the thought leader as an individual, and are not attributed to CeFPro or any particular organization.
What are the ways to deploy machine learning models in transition from transaction monitoring and why is it important?
The dynamic change in socio, political and geographical environment poses extremely complex challenges in keeping the transaction rules up to date and accurate. Traditional rules-based tools are deterministic, and the parameters are added as duct-tape and bandage method. These types of approaches soon become very difficult to maintain. The customer behaviours are rapidly changing, it is inevitable that the compliance programs need to shift from reactive to proactive strategies.
Modern machine learning algorithms deployed in transactional monitoring programs capture a large amount of data on similar transactions. They utilize the experience and behaviours of the outcomes of those transactions and dynamically assigns the risk for the new transactions. These risk scores can help to proactively identify the propensity for fraud. This dynamic risk scoring at transaction level is a huge advantage for AML programs and provide a leap from static rules based transactional monitoring programs.
There are many different types and levels of algorithms that can be deployed. The traditional statistical models such as regression and decision trees and relatively easy and widely used in AML programs. But due to the increase in the computing power, more sophisticated techniques such as random forest, neural network etc. are being increasingly adopted. These sophisticated ML models bring the real time risk at the POS.
By assigning the risk score to the transactions in real time, the compliance team can be able to optimize the resources. They can be able to streamline or automate investigations for low-risk transactions and prioritize the investigation resources on high-risk transactions. This helps in targeted investigation based on the risk score and improve the success rate per investigation.
How can false positives be reduced with the use of an automated program?
False positives cannot be fully eliminated in any AI/ML model. In other words, no model can be 100% perfect. The goal is to understand the business consequences of the false positive rate, and, to minimize them to a level that we can absorb the residual FP.
Traditional models lack the feedback loop. A well developed, automated ML/AI based AML program, not only provides the risk propensity of a transaction or customer or entity, but also incorporates the outcomes data and feeds it into the model. These outcomes will be used to finetune the Machine Learning algorithm and continuously enhance the accuracy of the program. This will help in dramatically reduce the false positive rate.
Automated AI and Machine Learning programs uses customer and transaction data and identifies true positives based on the history of the outcome. When this data is subjected to an investigation and identify any false positives, those false positive data and the underlying reasoning (or root cause data) is fed back as inputs to the next version of the model. These iterative processes use the root cause data along with other features and constantly refine the model on every iteration, thus minimizing the false positive rate.
The key is to establish and operationalizing the process of consuming the outcomes data and incorporating into the new models.
How do we measure the success of the technology driven AML program from data science perspective?
There are few different methodologies in measuring the program effectiveness. They vary in complexity in design and execution. Some provide a directional insight if the program is working or not while the other strategies provide a scientific evidence on the impact of the monitoring process & technologies on the AML program effectiveness.
The easiest and less complex one is a survey based method where the data on the perception on technology is collected to provide the qualitative impact of technology driven program’s success. Since the performance of technology is based on the survey data, this is highly perceptional/subjective.
The other methods are to look on the specific AML metrics before the implementation of technology and compare those with the metrics after the technology is implemented. This is called a pre-post comparison, where the outcomes (example, average frequency of fraudulent activities in a given time) are compared before and after the introduction of technology. Though this method is more effective than the qualitative survey based approach, the caveats of this type of analysis include, there could be a lot of environmental changes that could impact on the results. In other words, since the comparison is based on two different time points, any increase or decrease of the outcome cannot be fully attributed to the introduction of technology. The other factors such as novel fraudulent techniques by the fraudsters, geographical risk ratings and so on can influence the outcome.
To counter these limitations, the 3rd methodology that can be used is the longitudinal & cross-sectional analysis. With these types of outcomes analysis, the analyst compares two parallel AML programs one with rules based and other with AI/ML based (technologically advanced programs) on the same timeline. With the use of statistical methods the rules based programs and model based programs are compared and the difference in metrics/outcomes are measured if they differ significantly or not. Also, the difference is attributed to the technology alone by adjusting the bias due to anything other than the technology/ML model. These types of techniques can give the compliance officers a high confidence if the technology is helping them to address the fraud or if there is a need to tweak the program.