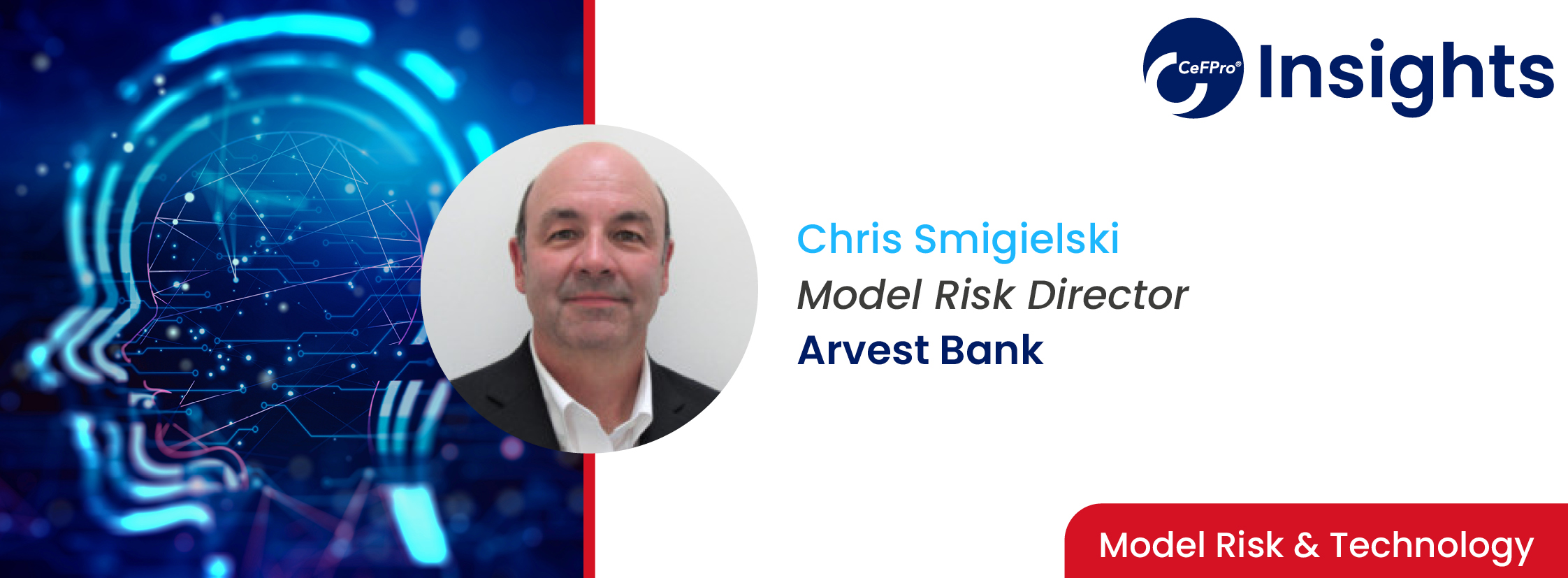
Inclusion of operational risks under model risk including cyber risk and fraud models
Chris Smigielski, Model Risk Director, Arvest Bank
Below is an insight into what can be expected from Chris’ session at Advanced Model Risk USA 2023.
The views and opinions expressed in this article are those of the thought leader as an individual, and are not attributed to CeFPro or any particular organization.
Why is expansion of model risk to include AI and machine learning important?
Technological and analytical advances are contributing to increased model complexity and use. Artificial intelligence (AI), including Machine Learning (ML), is used in a variety of ways. AI is broadly defined as the application of computational tools to address tasks traditionally requiring human analysis. Examples of AI uses in banks include fraud detection and prevention, marketing, chatbots, credit underwriting, credit and fair lending risk management, robo-advising (i.e., an automated digital investment advisory service), trading algorithms and automation, financial marketing analysis, cybersecurity, Bank Secrecy Act/anti-money laundering (BSA/AML) suspicious activity monitoring and customer due diligence, robotic process automation, and audit and independent risk management.
Some AI and ML solutions may meet the definition of a model as defined in the MRM Supervisory Guidance (FRB SR 11-7). While AI outputs are not always quantitative in nature, AI is typically based on complex mathematical techniques, which is categorically within the purview of model risk management . Regardless of how AI is classified (i.e., as a model or not a model), the associated risk management should be commensurate with the level of risk of the function that the AI supports.
AI and ML present new risks to the organization, but the risk model governance framework should be able to adapt to monitor and manage this change. These risks should still be managed by the business who should have an appropriate control framework to monitor performance. The first line always needs to understand and mitigate the risks so that customers and the business are protected but the increased adoption of AI/ML as models, model components, and tools is presenting a growing need for model risk management to expand their framework to govern the increased deployment of AI/ML applications across financial services.
How do validation requirements differ for non-financial risk models including cyber validation?
Validation approaches and techniques have adapted to the changing model landscape since SR 11-7 guidance was issued. In very general terms, financial models drew the most interest at that time because of their direct impact on financial statements and management decision-making. That was on the heels of the financial crisis over a decade ago. Since then, model inventories have broadened to include much more than just financial models. The tools and techniques to validate the newly added models are somewhat different because we are not calculating a quantitative result in some cases, so our validation playbook is more extensive. Process verification is key if the output is not a mathematical result as in some fraud monitoring models, for example. A newer aspect of the playbook is addressing AI/ML models and testing data for fairness, bias, and disparate impact, which is obviously a broader risk issue than quantitative accuracy. ‘Explainable AI’ is a phrase repeated quite often because lack of transparency and explainability can be interpreted as higher risk or higher uncertainty. To achieve explainable AI, you may need to implement techniques and methods that previously may not have been used by your MRM team.
According to McKinsey & Company;
“Cybersecurity solutions are required to fulfill a set of objectives including detection and prevention of intrusions, data and messaging security, and access management. A range of solutions from advanced analytics (for example, ML) to rule-based approaches (for instance, expert-driven nonmodels) can be leveraged to fulfill these objectives. As an example, ML solutions play a key role in detecting and preventing intrusions and denial of service attacks. Similarly, analytical solutions are leveraged by banks to ensure data and messaging security and provide overall response to end point threats. On the other end of the spectrum, rule-based approaches are leveraged by banks to implement controls for managing the Internet of Things (IoT) and preventing fraud from transactions. Additionally, qualitative, expert-based approaches are used for identity and access management, which are important since the introduction of shared data repositories and a cloud-connected world.”
The sheer number and variety of solutions deployed within cyber solutions requires a more comprehensive validation approach for cybersecurity models which would be very different than perhaps validating a credit decisioning model. A more comprehensive validation approach would evaluate conceptual soundness, thoroughness of first line assessment testing, and the vigorousness of the governance and controls around the model. Specific areas of interest would include input data appropriateness, evaluation of methodology including assumptions and limitations, testing done on model output including fairness/bias testing, evaluating the quality of model implementation including controls and report generation, and ongoing model governance by the first line which would include performance monitoring and vendor management.
Why is it important to understand nuances and requirements across domains when implementing new risk models?
The OCC has defined eight categories of risk for bank supervision purposes: credit, interest rate, liquidity, price, operational, compliance, strategic, and reputation. These risks are not mutually exclusive. Any product or service may expose a bank to multiple risks. Risks may also be interdependent, positively or negatively correlated, or may have concentrations that can significantly elevate risk. Concentrations may accumulate within and across products, business lines, geographic areas, countries, and legal entities.
Model use can affect all eight categories of risk. The use of models can increase or decrease risk in each risk category depending on the models’ purpose, use, and the effectiveness of any relevant model risk management. Conceptually, model risk is a distinct risk that can influence aggregate risk across all risk categories. Model risk can increase due to interactions and dependencies among models, such as reliance on common assumptions, inputs, data, or methodologies.
How do you see the inclusion of non-financial risk within model risk developing over the next 5 years?
Model Risk guidance initially targeted models that produce quantitative estimates or output that is quantitative in nature, which was most likely found in in Credit, Interest Rate, Liquidity, & Price risks. Updated expectations also find model risk impacting Operational, Compliance, Strategic, and Reputation risks as well. Models, tools, and algorithms used across the risk appetite introduce risk because of the consequences they may present if they are wrong.
As a single example, the OCC Booklet published in August 2021 explains that Operational risk can increase when the information technology (IT) environment supporting the bank’s models does not have appropriate internal controls. Security weaknesses, including poorly constructed application program interfaces (API’s) and weaknesses in the controls for the access, transmission, and storage of sensitive customer information, could expose a bank to increased operational risk. Weak or lax controls can compromise the confidentiality or integrity of sensitive customer data. Third-party risk management weaknesses related to a bank’s use of third parties providing models or related products and services could increase operational risk, particularly when management does not fully understand a third-party model’s capabilities, applicability, and limitations. New technologies, products, and services, such as AI and data aggregation, can increase third-party access to banks’ IT systems. Poorly drafted contracts could increase operational risk. Important considerations include the ability of the third party to resell, assign, or permit access to the bank’s data and IT systems to other entities and how the data will be transmitted, accessed, and used.
Over the next five years, digital transformation along with increased adoption of AI and ML solutions will drive an evolution of model risk management from ‘model centric’ governance to include a dimension of ‘algorithmic complexity’, including how data is used and treated for these solutions.