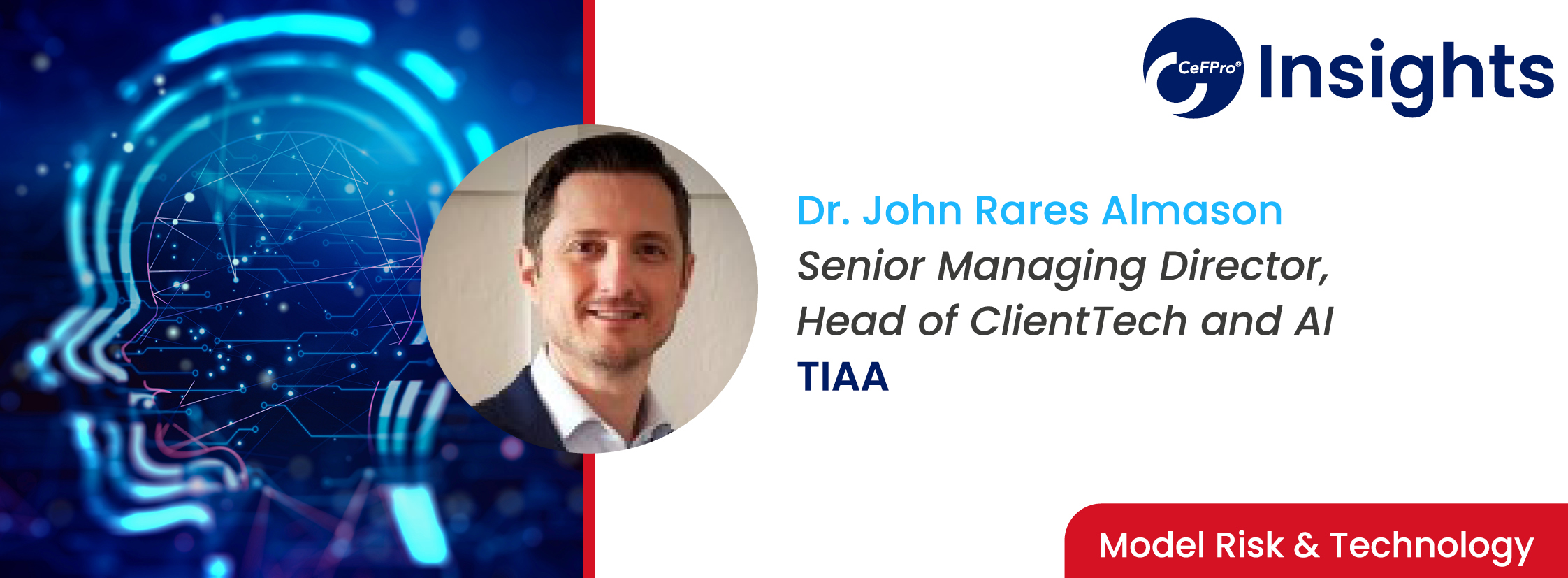
Ensuring generative AI tools for use within AI applications are used ethically and responsibly
Dr. John Rates Almasan, Senior Managing Director, Head of ClienTech and AI, TIAA
Below is an insight into what can be expected from John’s participation at CeFPro’s Digital Banking Congress.
The views and opinions expressed in this article are those of the thought leader as an individual, and are not attributed to CeFPro or any particular organization.
What can financial institutions do to ensure that generative AI applications are used ethically and responsibly?
Financial institutions have a pivotal role in ensuring the ethical and responsible deployment of generative AI applications. Their responsibility encompasses establishing robust governance frameworks and comprehensive policies that encompass transparency, accountability, and fairness. This entails the initial implementation and the continuous monitoring and reassessment of AI systems. Furthermore, instituting regular audits, assessments, and compliance checks helps maintain the integrity of AI-driven processes.
To cultivate an ethical AI environment, financial institutions should prioritize comprehensive training programs that equip employees with an understanding of ethical AI practices. This education extends to the highest executive level, fostering a culture of awareness and responsibility from top to bottom. Collaborations with AI ethics experts and fostering partnerships with organizations dedicated to responsible AI can provide invaluable insights and guidance.
As financial institutions emphasize the ethical dimensions of generative AI, they are poised to build lasting trust with both customers and stakeholders. Demonstrating a commitment to minimizing risks and mitigating biases bolsters their reputation and credibility in an increasingly AI-driven landscape. By adhering to these principles, financial institutions position themselves as leaders in the ethical adoption of generative AI.
In what ways can large language models support and enhance a user’s journey?
The influence of large language models on enhancing user experiences is both transformative and far-reaching. These models offer personalized recommendations that cater to individual preferences, enabling users to discover content tailored to their interests. Furthermore, their capacity to tackle intricate inquiries with comprehensive responses saves time and enhances user satisfaction.
Beyond text-based interactions, large language models empower users by enabling them to draft emails and generate content efficiently. This feature is especially valuable for professionals, allowing them to streamline their communication efforts. The ability of these models to comprehend and produce natural language makes interactions more conversational and human-like, fostering a stronger sense of engagement.
Large language models act as linguistic bridges in the global landscape, facilitating communication across language barriers. This not only enriches cross-cultural exchanges but also aids in expanding businesses’ reach to international markets. Moreover, the impact extends to customer service, where these models offer swift and accurate responses, enhancing overall satisfaction.
Can you describe the importance of having large volumes of accurate data when using generative AI?
Data’s role in shaping the efficacy of generative AI cannot be overstated. The models draw insights from the patterns and nuances embedded within the data they are trained on. High-quality data is the foundation upon which these models build their capabilities. Enriched by vast volumes of accurate data, these models can grasp an extensive array of contexts and intricacies, resulting in more precise and meaningful outputs.
The significance of accurate data cannot be separated from the importance of data diversity. A diverse dataset ensures that the models gain exposure to a variety of scenarios, ultimately leading to better generalization and adaptability in real-world contexts. Moreover, the use of accurate data safeguards against the propagation of misinformation, reinforcing the models’ reliability and trustworthiness.
Acquiring and curating high-quality data demands meticulous attention, as data quality directly influences the models’ performance. Strategies involving data augmentation, rigorous data cleaning processes, and regular updates contribute to a robust foundation for generative AI systems. In essence, data is the lifeline that nurtures the growth and accuracy of these models.
From your experience, what are the key areas that generative AI can help improve within banking?
Reflecting on my experiences, the transformative impact of generative AI in the banking sector is evident across several vital domains. Among these, customer service emerges as a prominent beneficiary. By seamlessly addressing customer inquiries and providing personalized recommendations, generative AI enhances the overall customer experience. This personalized touch fosters customer loyalty and augments the institution’s reputation for customer-centricity.
In risk management and fraud detection, generative AI’s capabilities are paramount. These systems analyze vast datasets in real-time, identifying irregular patterns and anomalous activities. This advanced monitoring plays a pivotal role in minimizing potential threats and safeguarding the financial ecosystem. Moreover, the efficiency gains garnered from automating back-office operations significantly optimize resource allocation and reduce operational costs.
The prospect of innovation-driven transformation extends to customer engagement. Generative AI can simulate scenarios, allowing customers to make informed financial decisions. This forward-looking approach empowers customers to anticipate potential outcomes, contributing to more confident decision-making.
How do you see generative AI, such as ChatGPT evolving the banking landscape?
The disruptive potential of generative AI, typified by entities like ChatGPT, stands poised to revolutionize the banking sector. At its core, this technology promises a paradigm shift in customer interactions. Conversations become personalized and human-like, enabling users to have their queries addressed promptly and efficiently. Beyond basic queries, customers can receive tailored financial advice and simulate diverse scenarios, leading to more informed decisions.
Furthermore, the implications for risk management and fraud prevention are significant. Generative AI’s ability to analyze vast data streams in real-time equips financial institutions with a dynamic defense against threats. Anomalies can be rapidly identified, bolstering security measures, and fostering a safer financial landscape.
As this technology evolves, its potential for innovation is boundless. The efficiency gains realized through automation in routine tasks extend to more complex processes like investment analysis and portfolio optimization. With the power of generative AI, financial institutions can optimize their operations, make better-informed decisions, and ultimately redefine how banking services are delivered. This evolution transcends transactional boundaries, promising a future where financial interactions are efficient, deeply engaging, and transformative.